Implementing one-way customer traffic at retail stores can dramatically reduce COVID transmission: Study
Feb 27, 2021
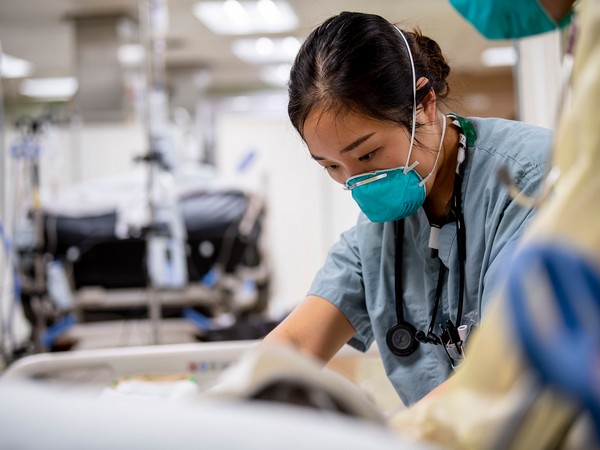
New Delhi [India], February 27 : Researchers in a study suggests that implementing one-way customer traffic at retail stores can dramatically reduce COVID-19 transmission. The study was conducted by Robert A. Shumsky, Laurens Debo, Rebecca M. Lebeaux, Quang P. Nguyen and Anne G. Hoen.
The study was published by Proceedings of the National Academy of Sciences.
"To reduce the transmission of COVID-19, many retail stores use one-way aisles, while local governments enforce occupancy limits or require "safe shopping" times for vulnerable groups," read the study.
To assess the value of these interventions, the study formulates and analyzes a mathematical model of customer flow and COVID-19 transmission. The study finds find that the value of specific operational changes depends on how the virus is transmitted, through close contact or suspended aerosols. If the transmission is primarily due to close contact, then restricting customers to one-way movement can dramatically reduce transmission. Other interventions, such as reductions in customer density, are effective at a distance but confront store operators with trade-offs between infection rates and customer flow.
The study examines how operational changes in customer flows in retail stores affect the rate of COVID-19 transmission. They combine a model of customer movement with two models of disease transmission: direct exposure when two customers are in close proximity and wake exposure when one customer is in the airflow behind another customer.
They find that the effectiveness of some operational interventions is sensitive to the primary mode of transmission. Restricting customer flow to one-way movement is highly effective if direct exposure is the dominant mode of transmission. In particular, the rate of direct transmission under full compliance with one-way movement is less than one-third of the rate under two-way movement.
Directing customers to follow the one-way flow, however, is not effective if wake exposure dominates. The study finds that two other interventions--reducing the speed variance of customers and throughput control--can be effective whether direct or wake transmission is dominant.
The study also examines the trade-off between customer throughput and the risk of infection to customers, and the study shows how the optimal throughput rate drops rapidly as the population prevalence rises.
By integrating knowledge about virology, epidemiology, and the physical flow of customers in retail stores, the study develops and analyze a model that connects the biology of COVID-19 with operational interventions to reduce the spread of the disease. The model calculates the disease transmission rate in a retail environment and determines how the rate depends on the customer flow policy (one-way versus two-way), the travel speed distribution, and store size. The study find that one-way restrictions are useful for reducing direct transmission but have no effect on wake transmission. Eliminating speed variability can reduce or even eliminate direct transmission when traffic is one-way but can only reduce (but not eliminate) transmission in two-way traffic. Finally, the study shows that the optimal admission rate to a store falls as the disease prevalence rises and the ratio of profit to infection cost falls.
The study also calibrates our model using published epidemiological data. For a medium-sized retailer in an area with a relatively high prevalence of COVID-19, our model predicts a total transmission rate (via direct and wake exposure) of 0.33 infections per day. Complete customer compliance with one-way flow reduces the direct transmission rate by 70%, while the partial compliance observed in one store reduces the transmission rate by 11 per cent. The study also compares the impact of direct and wake transmission by first defining a ratio between the wake and direct transmission probabilities for a single encounter between an infectious and a susceptible customer. Given two-way traffic, if this ratio is 200%200% or more, then wake exposure dominates direct exposure in its contribution to virus transmission. When retailers can control customer throughput, the optimal throughput drops significantly as COVID-19 prevalence increases up to 1%. Because estimates of some parameters vary substantially from region to region and will change over time, the study conduct sensitivity analysis to assess the robustness of our findings.
The study discusses some of our modeling assumptions and possible extensions. In the model of customer flow, the study ignores variation in both the speed and path of each customer, e.g., stopping to examine and pick products off shelves. Adding this additional variability would increase the number of customer interactions and direct exposure, although quantifying its impact and understanding the impact on wake exposure would require a more complex analytical model and/or simulation. The study also assumes Poisson arrivals, a reasonable assumption during short periods in many retail areas, but not a reasonable assumption in other contexts such as school hallways, where a fluid model may be more appropriate.
In addition, the study assumes that wake exposure ends as soon as an infectious customer leaves the area. This is a simplification, for infectious aerosols may remain in the air after customers depart (20). If the area is large, however, and if customers spend a significant amount of time on their journey, then the impact of edge effects when customers leave the area is small. For the numerical experiments described above, these edge effects are not significant.
Our model focuses on customer movement through store aisles and ignores interactions among customers and employees. Front-line workers for essential retailers, in particular, can face a high risk of exposure (47). Cashiers, for example, face both direct and wake exposure in checkout areas. Many stores now enforce social distancing standards around these areas and provide guidance such as floor stickers to indicate spacing between customers. While this can nearly eliminate direct exposure, wake exposure would be a concern around checkout areas. Incorporating a queuing model that includes customer movement and airflow may be a useful extension.
The specific infection rates predicted by our model depend upon many environmental variables, including ventilation systems and the proportion of customers who use masks. Given this uncertainty, the model highlights where gaps in the scientific knowledge of COVID-19 transmission need to be filled so that the study may redesign service operations to reduce spread. In particular, the effectiveness of these interventions is highly dependent on whether the virus is transmitted through heavy droplets or aerosols. In addition, the model may be adapted to analyze COVID-19 transmission in other service settings such as restaurants, airplanes (supplement to ref. 48), and hotels in which customer flow can be regulated.